Filter results
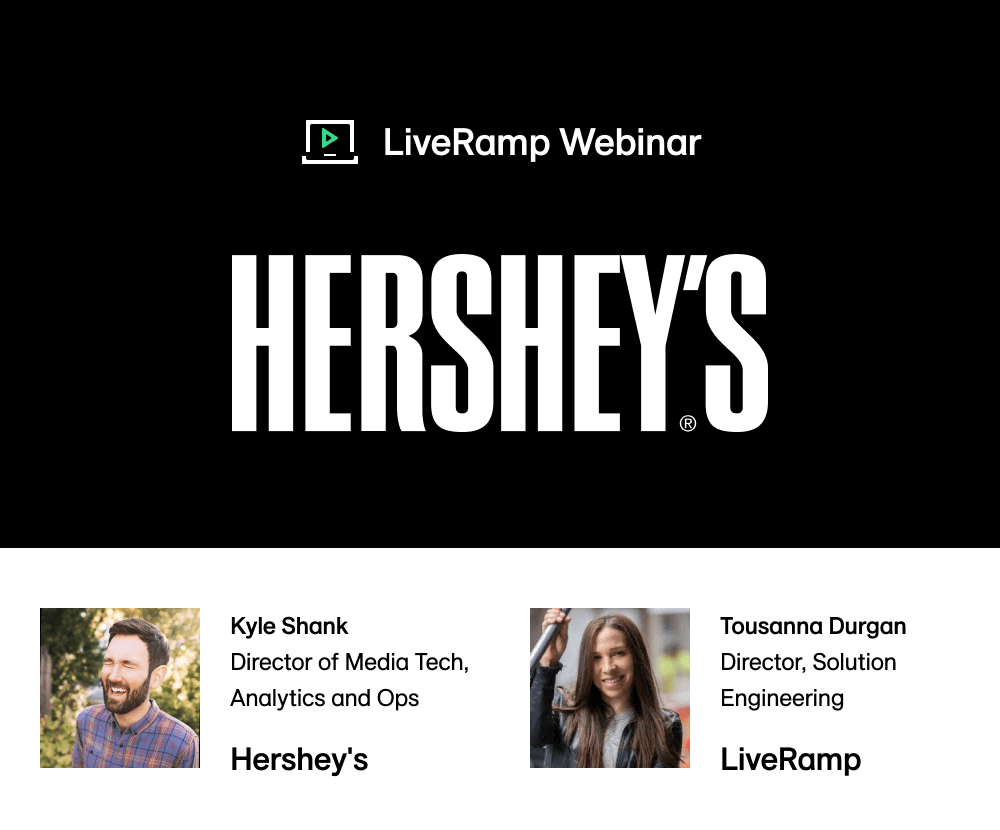
Sweet Success: Hershey’s Recipe to Improve Advertising Effectiveness
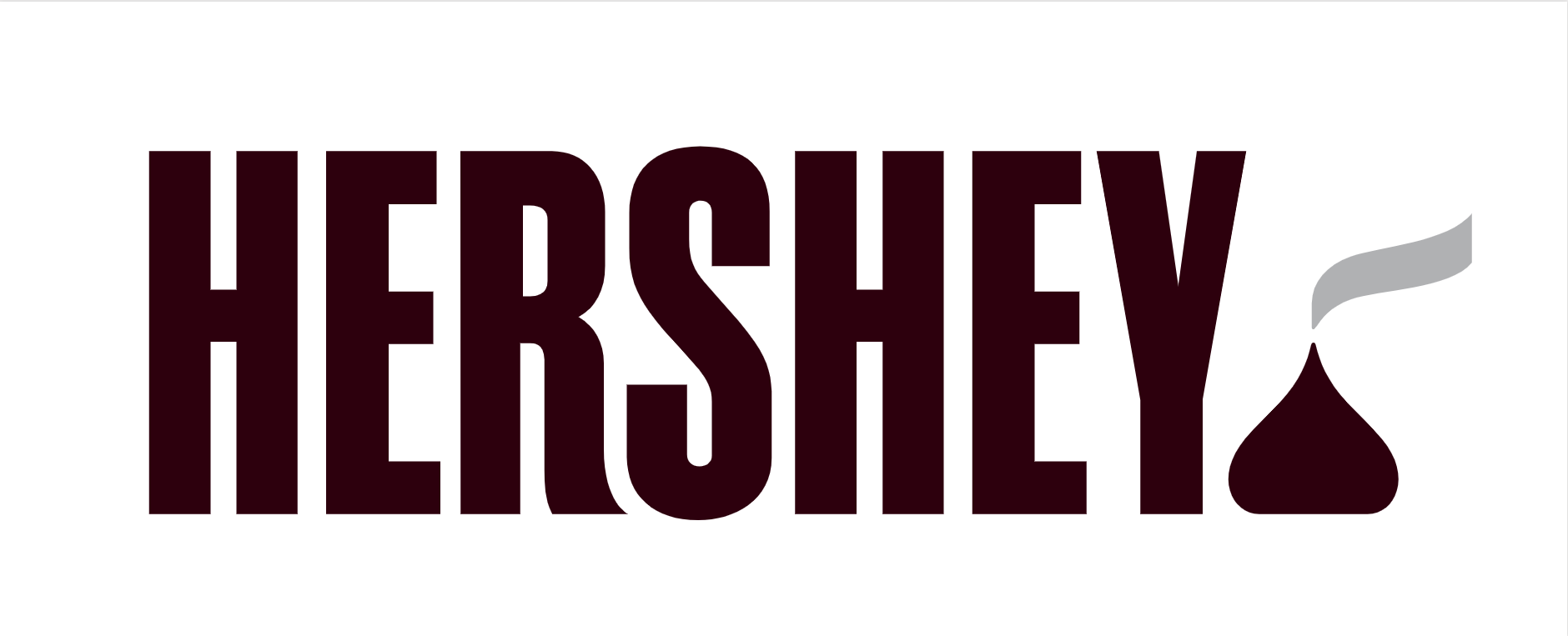
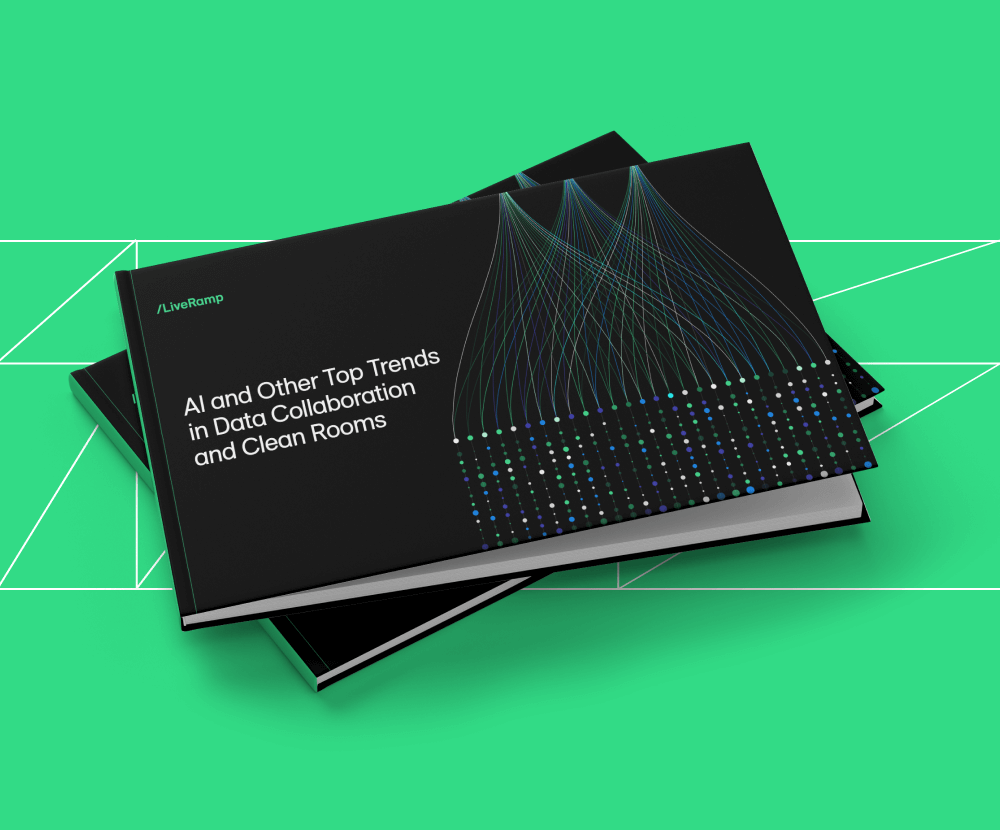
AI and Other Top Trends in Data Collaboration and Clean Rooms
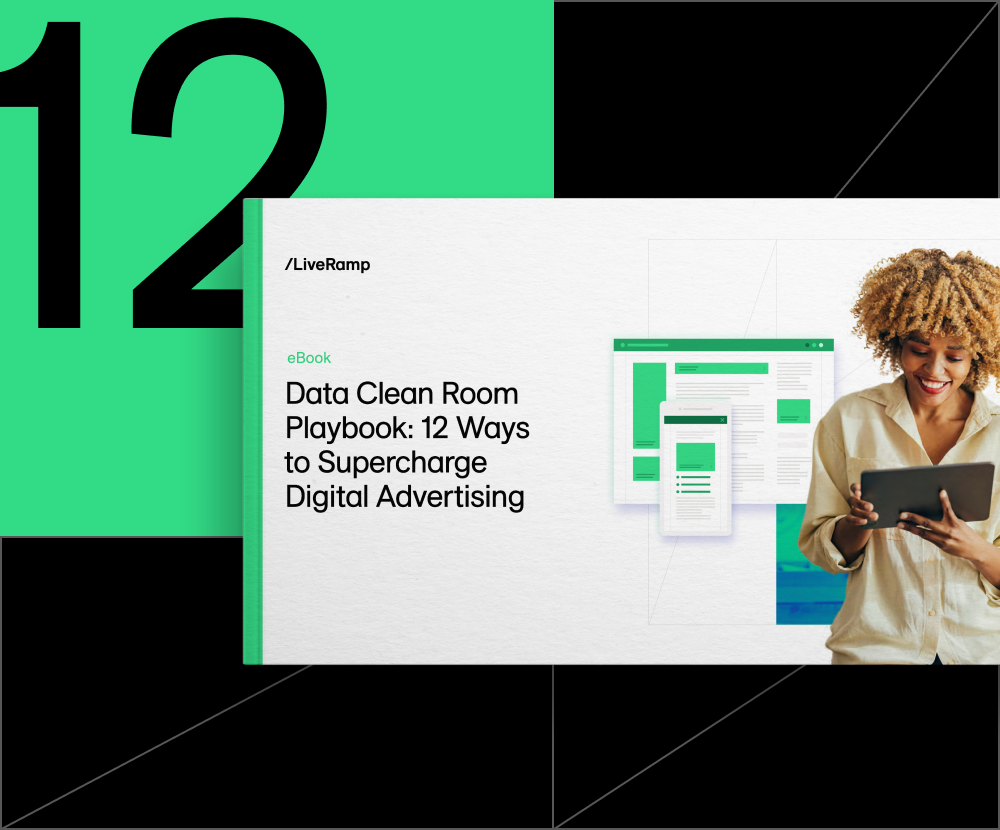
Data Clean Room Playbook: 12 Ways to Supercharge Digital Advertising
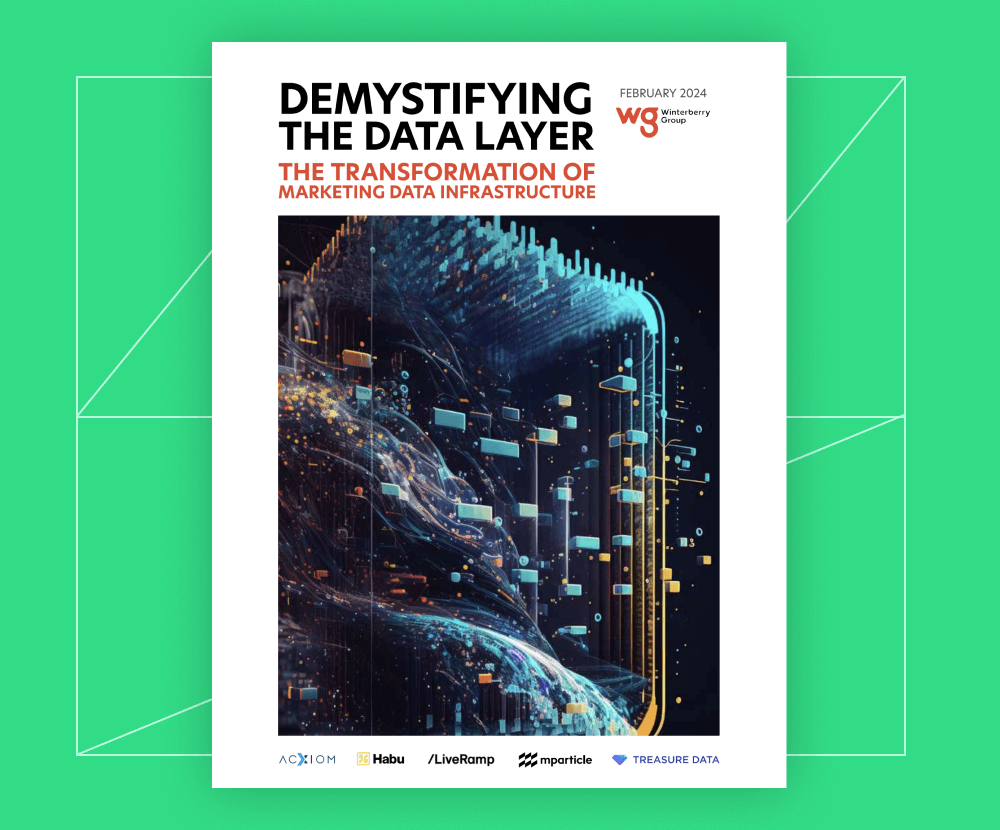
Winterberry Report: Demystifying the Data Layer: The Transformation of Marketing Data Infrastructure
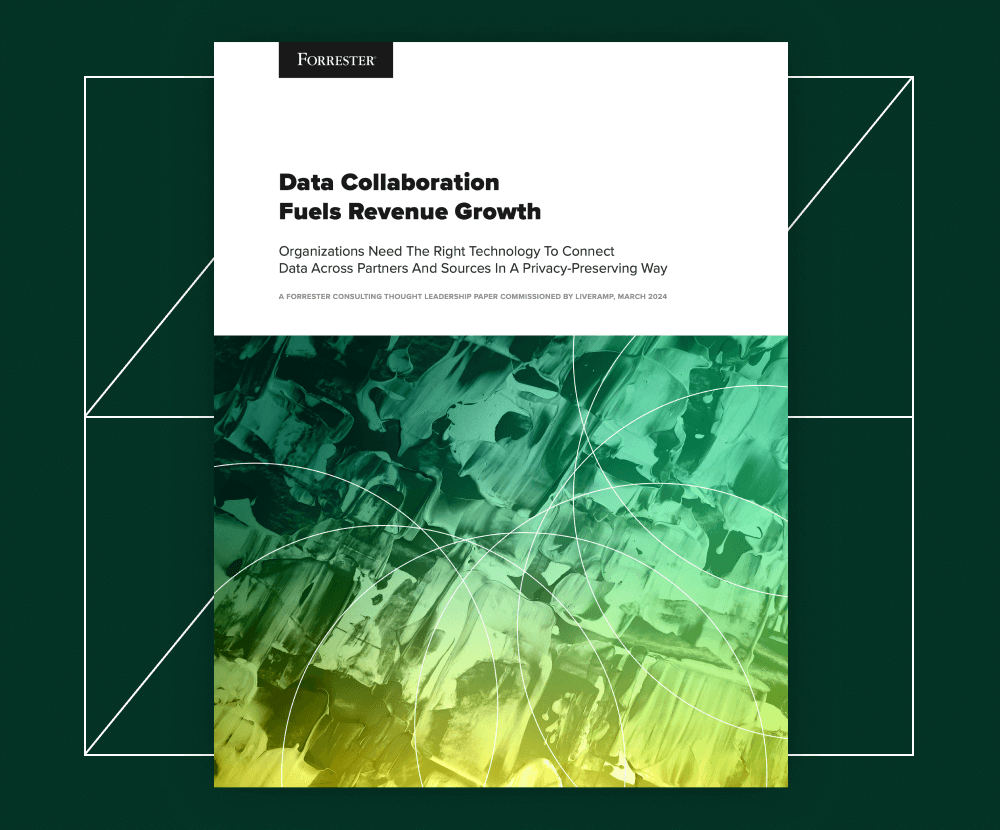
Research Study: Data Collaboration Fuels Revenue Growth
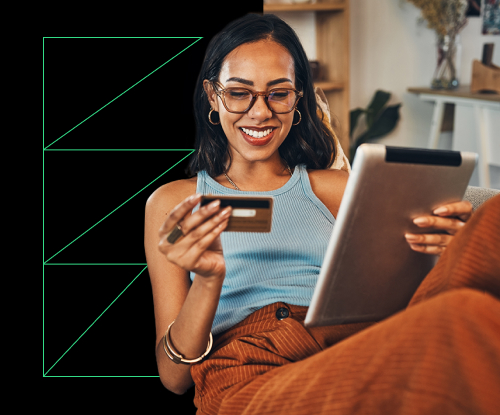
How to Improve Marketing Performance at Financial Services Brands in 2024
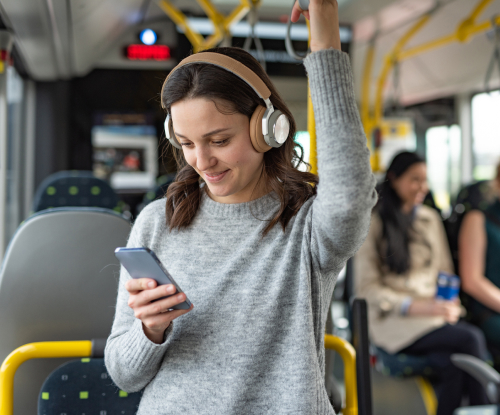
Maximizing Mobile Ad Success: the Nimbus and LiveRamp Authenticated Traffic Solution Strategy
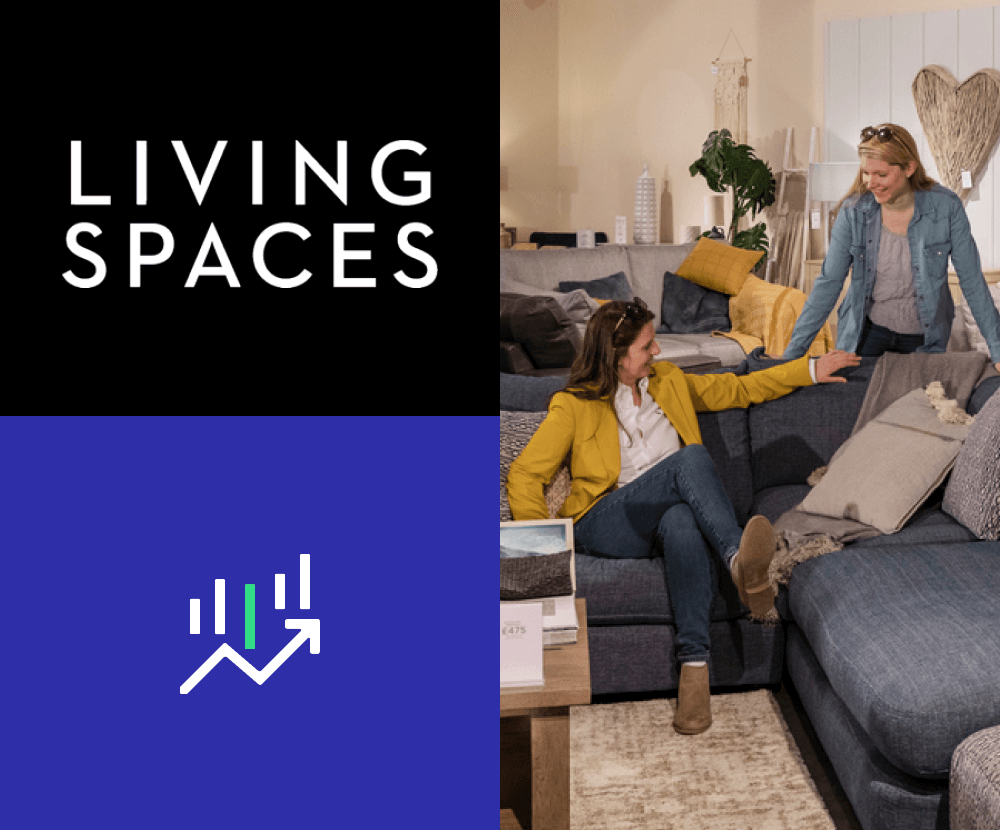
How Living Spaces Makes Smarter Marketing Investments with Data Collaboration
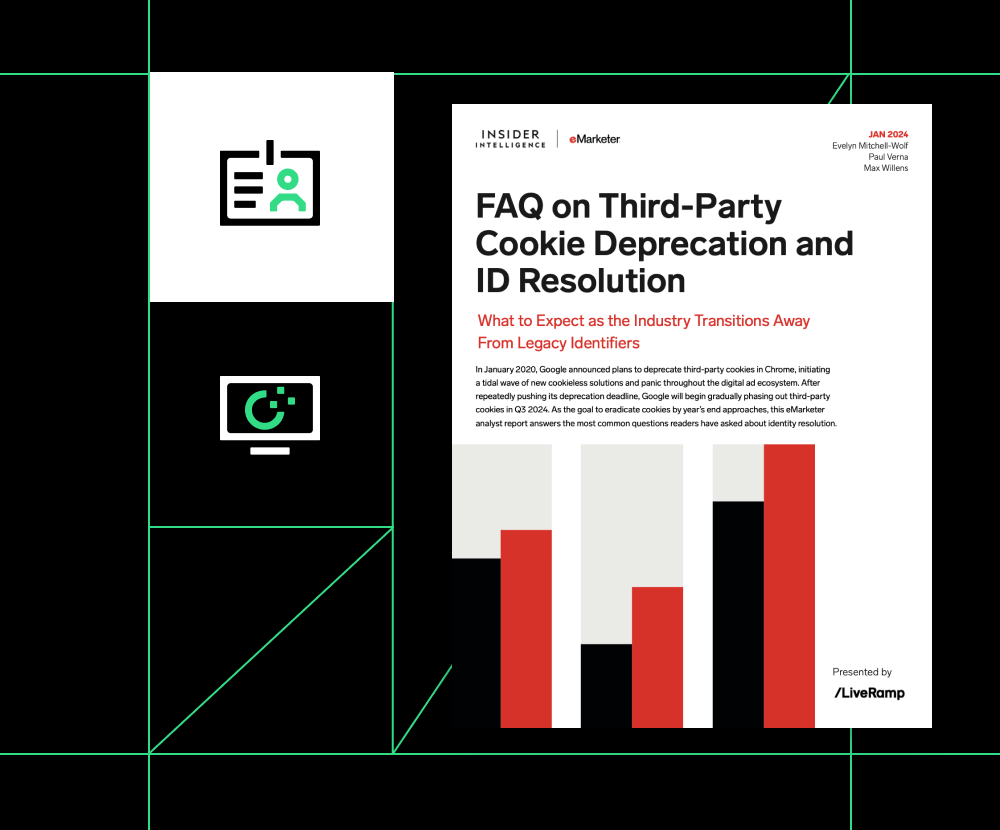
eMarketer Analyst Report: FAQ on Third-Party Cookie Deprecation and ID Resolution
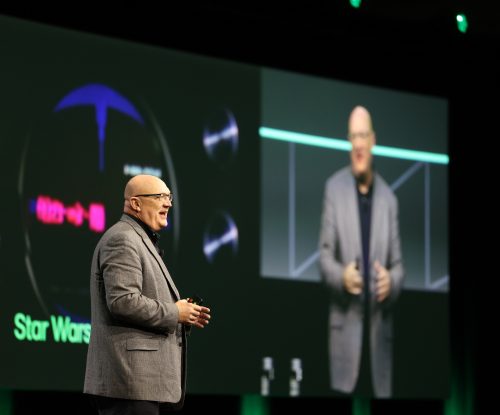
Moments That Change Everything
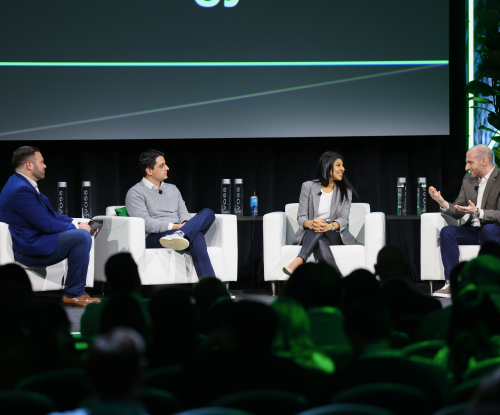
Data Collaboration: Supercharging Your Data Strategy
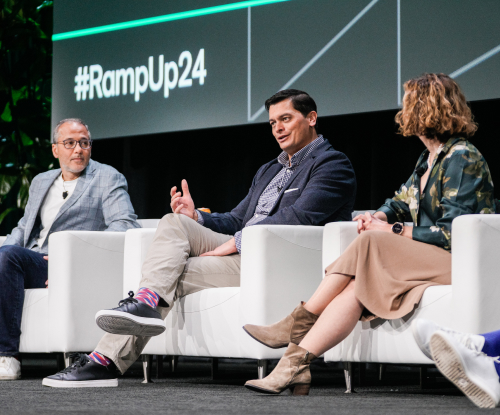